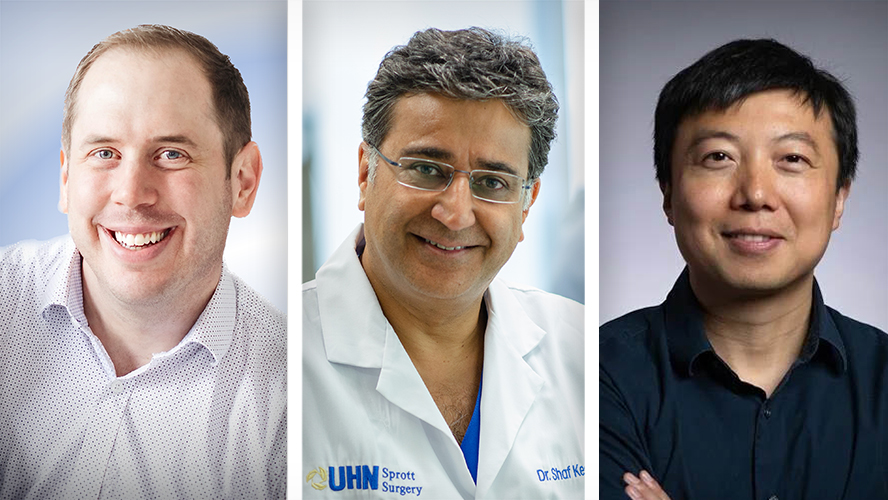
A new study from researchers at Toronto General Hospital Research Institute (TGHRI) has identified a way to predict and guide transplant physicians on donor lung suitability and transplant outcomes using artificial intelligence (AI) and machine learning tools.
Ex vivo lung perfusion (EVLP) is a medical technique, invented at UHN, in which a donated lung is preserved outside of the body so that it can be assessed and treated prior to transplant. This process helps to ensure that the lung is healthy and functioning properly before being transplanted into a patient.
“Although EVLP has improved the number of transplant procedures conducted, this increase still falls significantly short of the growing number of individuals added to the transplant waiting list each year—a problem that has been compounded by the pandemic,” says Dr. Shaf Keshavjee, Director of the Latner Thoracic Surgery Research Laboratories, Director of the Toronto Lung Transplant Program within the Ajmera Transplant Centre and Sprott Department of Surgery, Senior Scientist at TGHRI and Chief of Innovation at UHN.
“Additionally, the assessment of lungs undergoing EVLP is largely subjective. There are no standardized guidelines that can accurately determine which organs are suitable for transplant.”
While AI and machine learning tools have begun to make an impact on clinical decision-making, the use of AI for organ transplantation has not yet been studied.
“We wanted to understand whether AI could aid clinicians in determining whether a donor lung was suitable for transplant,” says Dr. Bo Wang, Chief AI Scientist at UHN and Scientist at TGHRI. “To do this, we utilized a machine learning program called Extreme Gradient Boosting (XGBoost), which studies large sets of data to make predictions using an ensemble of decision trees—a series of questions that the model asks about the data.”
Using the XGBoost technique, the team developed a model, termed InsighTx, that uses data from EVLP assessments and donor features to predict which lungs are suitable for transplantation and importantly—patient outcome.
“We analyzed data from 725 EVLP cases using InsighTx,” says Dr. Andrew Sage, Assistant Scientist within the Toronto Lung Transplant Program, Ajmera Transplant Centre and at TGHRI. “We showed that the InsighTx AI model learned from this EVLP data and was able to predict which lungs were unsuitable for transplant and which transplants would have good outcomes for patients.”
Additionally, in a real-world evaluation of surgical decision making, lung suitability assessments made by a group of trained clinicians were compared with or without the aid of InsighTx. The results of this evaluation demonstrated that InsighTx increased the likelihood of getting it right—that is transplanting suitable donor lungs and not transplanting unsuitable donor lungs.
This study suggests that the impact of AI on transplantation rates could be dramatic and that an overall increase in safe organ utilization and transplant activity is plausible.
“This is the first evidence that an AI-guided approach could lead to a safe increase in the number of transplants performed following EVLP,” says Dr. Sage. “As the need for viable organs continues to grow, this knowledge would be a significant step forward in the field of organ transplantation.”
This work was supported by the Ajmera Transplant Centre, the Canadian Institutes of Health Research and UHN Foundation.
Dr. Bo Wang is a Tier 2 Canada Research Chair in Artificial Intelligence for Medicine. Drs. Sage and Wang are Assistant Professors at the University of Toronto. Dr. Keshavjee is a Professor at the University of Toronto.
Dr. Keshavjee serves as Chief Medical Officer of Traferox Technologies and receives personal fees from Lung Bioengineering, outside of this published work. Drs. Sage, Valero, Liu, Cypel, Wang and Keshavjee are inventors of patents related to this work.
Sage AT, Donahoe LL, Shamandy AA, Mousavi SH, Chao BT, Zhou X, Valero J, Balachandran S, Ali A, Martinu T, Tomlinson G, Del Sorbo L, Yeung JC, Liu M, Cypel M, Wang B, Keshavjee S. A machine-learning approach to human ex vivo lung perfusion predicts transplantation outcomes and promotes organ utilization. Nat Commun. 2023 Aug 9;14(1):4810. doi: 10.1038/s41467-023-40468-7. PMID: 37558674; PMCID: PMC10412608.
InsighTx uses data from EVLP and donors in order to make a decision on suitability for transplant. Data types include airway pressure, biomarkers of inflammation, donor body mass index and more. Image created with BioRender.com.