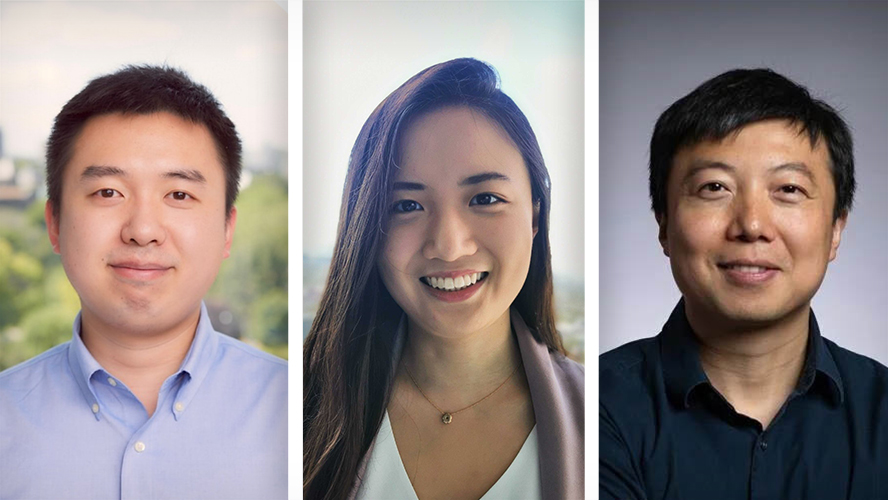
A research team at Toronto General Hospital Research Institute (TGHRI) has built an artificial intelligence (AI) learning model to understand complex biological interactions from large-scale datasets of the analysis of single cells.
Recent advancements in the study of the genes and gene expression patterns in single cells have provided a wealth of data that enables researchers to learn about cellular diversity, function, and how cells respond to various conditions. The use of a technique called single-cell RNA sequencing—a method that measures the levels of gene expression in each cell to determine how it functions—has led to the development of comprehensive data atlases.
“The large volume of sequencing data has created huge analytical challenges,” says Dr. Bo Wang, Scientist at TGHRI and senior author of the study. “To address this, we wanted to develop a foundation model to employ machine learning to decode and predict single-cell behaviours from sequencing data.”
A foundation model can be described as a giant database of information that is trained on a large number of diverse datasets and can be adapted for a variety of tasks. Language models, like chatGPT, are trained on text to learn patterns and meanings in language. Then, the model can be used to assist with tasks such as answering questions, summarizing text, or translating languages.
“While texts are made up of words, cells can be characterized by genes and the protein products they encode,” says Haotian Cui, doctoral student in Dr. Wang’s lab and co-first author of the study. “Using this principle, we developed a foundation model called scGPT (singe cell GPT) to examine single cell biology by pre-training on over 33 million cells.”
By training on a diverse dataset containing millions of cells from different tissues and conditions (i.e., cell types from 51 organs or tissues and 441 studies), scGPT has learned to understand patterns in gene expression and cell behavior and has been taught to create new information based on what it learned. Its main part uses special tools called transformer blocks to help it understand and process the data. After its initial training, its settings can be adjusted to make it work better with new information, which can be useful for various tasks.
The team found that scGPT is effective for tasks such as identifying cell types, predicting gene activity in cells, correcting batch effect errors in sequencing data, and uncovering important gene interactions that vary depending on the cell type or condition. This approach enhances the modeling of single-cell sequencing data and provides valuable insights into gene-gene interactions specific to different conditions such as cell states and gene expression disruptions.
“The release of scGPT models and workflows will be able to accelerate research in cellular biology and beyond, offering a standardized approach for analyzing single-cell omics—the profiling of single cells in various populations,” says Chloe Wang, co-first author of the study and doctoral student at TGHRI.
By leveraging the power of a pre-trained generative AI model, researchers hope to pave the way for innovative therapeutic strategies and deepen understanding of cellular processes.
“For the future, our goal is to make our model smarter and better at understanding how cells work in different situations,” adds Dr. Wang who is also Chief AI Scientist at UHN and co-lead of the UHN AI Hub.
Since the preprint of this study in May 2023 and the release of scGPT, it has significantly impacted the field, with over 13,000 installations and 55 citations before its official publication.
(L) scGPT was trained using single cell RNA-sequencing data from 33 million normal human cells from various organs. (R) Simplified workflow of scGPT. Images adapted from Cui et al., 2024, Nature Methods using BioRender.
This work was supported by funding from the Natural Sciences and Engineering Research Council of Canada, the Canadian Institute For Advanced Research AI Chairs Program, and the Peter Munk Cardiac Centre at the University Health Network.
Dr. Bo Wang is a Tier 2 Canada Research Chair in Artificial Intelligence for Medicine and an Assistant Professor at the University of Toronto.
Dr. Bo Wang is on the advisory board of Vevo Therapeutics and co-author Dr. Nan Duan is an employee of Microsoft and holds equity in the company.
Cui H, Wang C, Maan H, Pang K, Luo F, Duan N, Wang B. scGPT: toward building a foundation model for single-cell multi-omics using generative AI. Nat Methods. 2024 Feb 26. doi: 10.1038/s41592-024-02201-0. Epub ahead of print. PMID: 38409223.