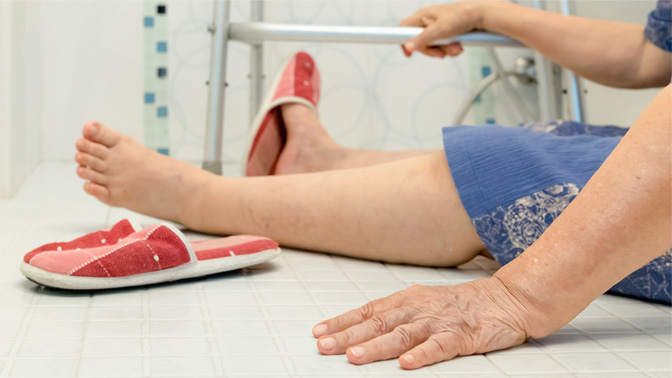
“I’ve fallen, and I can’t get up!” is a well-known catchphrase from a 1980s television commercial. The commercial advertised a pendant that could be used by the elderly to alert a dispatch service in the case of a medical emergency, such as a fall.
A limitation of this product is that the wearer of the pendant must be alert enough to report the need for medical attention; however, falls can cause many types of injuries that can leave people unable to communicate. While devices to automatically detect falls exist, many of them frequently trigger false alarms.
To improve the automated detection of falls, researchers have focused on teaching computers to identify falls by using data that is collected from sensors worn on the body; but, falls are a rare event, making it difficult to collect data on real life falls.
A recent study by TRI Scientist Dr. Babak Taati and his postdoctoral fellow Dr. Shehroz Khan aimed to address this issue from a new perspective: instead of using wearable sensor data to teach computers to identify falls, the researchers used the data to teach computers to identify normal behaviours such as standing, jogging/running and jumping—thus defining falls as an abnormal event. The researchers then tested the model with data sets gathered using wearable sensors.
Using computer simulations, their model performed better than traditional methods in differentiating normal from abnormal activities.
The team is now focused on further fine-tuning their computer-based model. “Although our model is still in its early stages, it is a crucial first step towards an accurate automated fall detection device,” says Dr. Taati. “Such a device could be especially useful for the elderly, who are at risk for developing serious medical problems as a result of falling.”
This work was supported by AGE-WELL, the Canadian Consortium on Neurodegeneration in Aging, the Toronto Rehabilitation Institute and the Toronto Rehab Foundation.
Khan SS, Taati B. Detecting unseen falls from wearable devices using channel-wise ensemble of autoencoders. Expert Syst Appl. 2017 Jun 15. doi: 10.1016/j.eswa.2017.06.011.