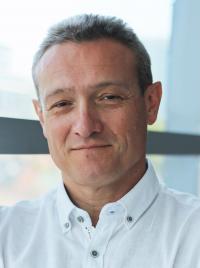
Dr. Igor Jurisica is a Senior Scientist at Krembil Research Institute, Professor at University of Toronto and Visiting Scientist at IBM CAS. He is also an Adjunct Professor at the School of Computing, Pathology and Molecular Medicine at Queen's University, Computer Science at York University, an adjunct scientist at the Institute of Neuroimmunology, Slovak Academy of Sciences and an Honorary Professor at Shanghai Jiao Tong University. Since 2015, he has also served as Chief Scientist at the Creative Destruction Lab, Rotman School of Management.
He has published extensively on data mining, visualization and cancer informatics, including multiple papers in Science, Nature, Nature Medicine, Nature Methods, J Clinical Investigations, J Clinical Oncology, and has over 12,299 since 2014, including 848 highly influential citations (SemanticScholar), and 13,795 lifetime citations (WOS) with h-index 52.
Dr. Jurisica has won numerous awards, including a Tier I Canada Research Chair in Integrative Cancer Informatics, the IBM Faculty Partnership Award (3-time recipient), and IBM Shared University Research Award (4-time recipient). He has been included in Thomson Reuters 2016, 2015 & 2014 list of Highly Cited Researchers, and The World's Most Influential Scientific Minds: 2015 & 2014 Reports. In 2019 he was included in the Top 100 AI Leaders in Drug Discovery and Advanced Healthcare list (Deep Knowledge Analytics).